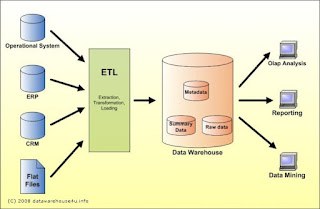
Source:
http://www.freewebs.com/testingtutorials/etltesting.htm
http://www.learn.geekinterview.com/data-warehouse/dw-basics/what-is-data-completeness.html
http://datawarehouse4u.info/index_en.html
What is ETL Testing?
ETL Testing:
During ETL application testing, we test for the following:
Data completeness. Ensures that all expected data is loaded.
Data transformation. Ensures that all data is transformed correctly according to business rules and/or design specifications.
Data quality. Ensures that the ETL application correctly rejects, substitutes default values, corrects or ignores and reports invalid data.
Performance and scalability. Ensures that data loads and queries perform within expected time frames and that the technical architecture is scalable.
Integration testing. Ensures that the ETL process functions well with other upstream and downstream processes.
User-acceptance testing. Ensures the solution meets users' current expectations and anticipates their future expectations.
Regression testing. Ensures existing functionality remains intact each time a new release of code is completed.
ETL testing validates that data is transformed correctly from OLTP to data
warehouse.
Validating the data transformed includes following main verification points:
1. Ensures that all expected data is loaded. Comparing record counts between source data loaded to the warehouse and rejected records.
2. Ensures that all data is transformed correctly according to design
specifications.
3. Ensures that the ETL application substitutes default values ignores
invalid data.
4. Validate correct processing of ETL-generated fields such as surrogate
keys.
5. Validate that data types in the warehouse are as specified in the design
and/or the data model.
6. Validate the referential integrity between tables.
Basics of Data warehousing:
In any data resource, it is essential to meet requirements of current as well as future demand for information. Data completeness assures that the above criterion is fulfilled.
Data completeness refers to an indication of whether or not all the data necessary to meet the current and future business information demand are available in the data resource.
It deals with determining the data needed to meet the business information demand and ensuring those data are captured and maintained in the data resource so they are available when needed.
A data warehouse has six main processes. These processes should be carefully carried out by the data warehouse administrator in order to achieve data completeness. The processes are as follows:
• Data Extraction – the data in the warehouse can come from many sources and of multiple data format and types with may be incompatible from system to system. The process of data extraction includes formatting the disparate data types into one type understood by the warehouse. The process also includes compressing the data and handling of encryptions whenever this applies.
• Data Transformation – This process include data integration, demoralization; surrogate key management, data cleansing, conversion, auditing and aggregation.
• Data Loading – After the first two process, the data will then be ready to be optimally stored in the data warehouse.
• Security Implementation – Data should be protected from prying eyes whenever applicable as in the case of bank records and credit card numbers. The data warehouse administrator implements access and data encryption policies.
• Job Control – This process is the constant job of the data warehouse administrator and his staff. This includes job definition, time and event job scheduling, logging, monitoring, error handling, exception handling and notification.
The measure of a data warehouse's performance depends on one of the factors pertaining to availability of useful data which is also an indication of the success of a business organization in reaching its own goals. All data can be imperfect in some fashion to some degree. It is the goal of the data warehouse manager to pursue perfect data which is consumed by the public resources without the need for creating appreciable value. The data warehouse manager and his staff should come up with strategies to be able to provide substantial accuracy and timeliness of data at a reasonable cost so as not to burden the company with extra expenses.
In most cases, data warehouses are available twenty four hours a day, seven days a week. So that comprehensive data is gathered, extracted, loaded and shared within the data warehouse, regular updates should be done. Parallel and distributed servers target for world wide availability of data so data completeness can be achieved with investing in high powered servers and robust software applications. Data warehouses are also designed for customer level analysis, aside from organizational level analysis and reporting. So flexible tools should be implemented in the data warehouse database to accommodate new data sources and support for metadata. Reliability can be achieved when all these are considered.
The success in achieving data completeness in a warehouse is not just dependent on the current status of the database and its physical set-up. At the planning stage, every detail about the data warehouse should be carefully scrutinized. All other frameworks of the data warehouse should also be carefully planned including the details of the business architecture, business data, business schema, business activities, data model, critical success factors, meta data, comprehensive data definition and other related aspects of organizational functions.
Having complete data can give an accurate guidance of the business organization's decision maker. With complete data, statistical reports will be generated with will reflect and accurate status of the company and how it is faring with the trends and patterns in the industry and how to make innovative moves to gain competitive advantages over the competitors.
Data warehouse Overview:
ETL process
ETL (Extract, Transform and Load) is a process in data warehousing responsible for pulling data out of the source systems and placing it into a data warehouse. ETL involves the following tasks:
- extracting the data from source systems (SAP, ERP, other oprational systems), data from different source systems is converted into one consolidated data warehouse format which is ready for transformation processing.
- transforming the data may involve the following tasks:
applying business rules (so-called derivations, e.g., calculating new measures and dimensions),
cleaning (e.g., mapping NULL to 0 or "Male" to "M" and "Female" to "F" etc.),
filtering (e.g., selecting only certain columns to load),
splitting a column into multiple columns and vice versa,
joining together data from multiple sources (e.g., lookup, merge),
transposing rows and columns,
applying any kind of simple or complex data validation (e.g., if the first 3 columns in a row are empty then reject the row from processing)
- loading the data into a data warehouse or data repository other reporting applications
Data Completeness
One of the most basic tests of data completeness is to verify that all expected data loads into the data warehouse. This includes validating that all records, all fields and the full contents of each field are loaded. Strategies to consider include:
Comparing record counts between source data, data loaded to the warehouse and rejected records.
Comparing unique values of key fields between source data and data loaded to the warehouse. This is a valuable technique that points out a variety of possible data errors without doing a full validation on all fields.
Utilizing a data profiling tool that shows the range and value distributions of fields in a data set. This can be used during testing and in production to compare source and target data sets and point out any data anomalies from source systems that may be missed even when the data movement is correct.
Populating the full contents of each field to validate that no truncation occurs at any step in the process. For example, if the source data field is a string (30) make sure to test it with 30 characters.
Testing the boundaries of each field to find any database limitations. For example, for a decimal (3) field include values of -99 and 999, and for date fields include the entire range of dates expected. Depending on the type of database and how it is indexed, it is possible that the range of values the database accepts is too small.
Data Transformation
Validating that data is transformed correctly based on business rules can be the most complex part of testing an ETL application with significant transformation logic. One typical method is to pick some sample records and "stare and compare" to validate data transformations manually. This can be useful but requires manual testing steps and testers who understand the ETL logic. A combination of automated data profiling and automated data movement validations is a better long-term strategy. Here are some simple automated data movement techniques:
Create a spreadsheet of scenarios of input data and expected results and validate these with the business customer. This is a good requirements elicitation exercise during design and can also be used during testing.
Create test data that includes all scenarios. Elicit the help of an ETL developer to automate the process of populating data sets with the scenario spreadsheet to allow for flexibility because scenarios will change.
Utilize data profiling results to compare range and distribution of values in each field between source and target data.
Validate correct processing of ETL-generated fields such as surrogate keys.
Validate that data types in the warehouse are as specified in the design and/or the data model.
Set up data scenarios that test referential integrity between tables. For example, what happens when the data contains foreign key values not in the parent table?
Validate parent-to-child relationships in the data. Set up data scenarios that test how orphaned child records are handled.
Data Quality
For the purposes of this discussion, data quality is defined as "how the ETL system handles data rejection, substitution, correction and notification without modifying data." To ensure success in testing data quality, include as many data scenarios as possible. Typically, data quality rules are defined during design, for example:
Reject the record if a certain decimal field has nonnumeric data.
Substitute null if a certain decimal field has nonnumeric data.
Validate and correct the state field if necessary based on the ZIP code.
Compare product code to values in a lookup table, and if there is no match load anyway but report to users.
Depending on the data quality rules of the application being tested, scenarios to test might include null key values, duplicate records in source data and invalid data types in fields (e.g., alphabetic characters in a decimal field). Review the detailed test scenarios with business users and technical designers to ensure that all are on the same page. Data quality rules applied to the data will usually be invisible to the users once the application is in production; users will only see what's loaded to the database. For this reason, it is important to ensure that what is done with invalid data is reported to the users. These data quality reports present valuable data that sometimes reveals systematic issues with source data. In some cases, it may be beneficial to populate the "before" data in the database for users to view.
Performance and Scalability
As the volume of data in a data warehouse grows, ETL load times can be expected to increase and performance of queries can be expected to degrade. This can be mitigated by having a solid technical architecture and good ETL design. The aim of the performance testing is to point out any potential weaknesses in the ETL design, such as reading a file multiple times or creating unnecessary intermediate files. The following strategies will help discover performance issues:
Load the database with peak expected production volumes to ensure that this volume of data can be loaded by the ETL process within the agreed-upon window.
Compare these ETL loading times to loads performed with a smaller amount of data to anticipate scalability issues. Compare the ETL processing times component by component to point out any areas of weakness.
Monitor the timing of the reject process and consider how large volumes of rejected data will be handled.
Perform simple and multiple join queries to validate query performance on large database volumes. Work with business users to develop sample queries and acceptable performance criteria for each query.
Integration Testing
Typically, system testing only includes testing within the ETL application. The endpoints for system testing are the input and output of the ETL code being tested. Integration testing shows how the application fits into the overall flow of all upstream and downstream applications. When creating integration test scenarios, consider how the overall process can break and focus on touch points between applications rather than within one application. Consider how process failures at each step would be handled and how data would be recovered or deleted if necessary.
Most issues found during integration testing are either data related to or resulting from false assumptions about the design of another application. Therefore, it is important to integration test with production-like data. Real production data is ideal, but depending on the contents of the data, there could be privacy or security concerns that require certain fields to be randomized before using it in a test environment. As always, don't forget the importance of good communication between the testing and design teams of all systems involved. To help bridge this communication gap, gather team members from all systems together to formulate test scenarios and discuss what could go wrong in production. Run the overall process from end to end in the same order and with the same dependencies as in production. Integration testing should be a combined effort and not the responsibility solely of the team testing the ETL application.
User-Acceptance Testing
The main reason for building a data warehouse application is to make data available to business users. Users know the data best, and their participation in the testing effort is a key component to the success of a data warehouse implementation. User-acceptance testing (UAT) typically focuses on data loaded to the data warehouse and any views that have been created on top of the tables, not the mechanics of how the ETL application works. Consider the following strategies:
Use data that is either from production or as near to production data as possible. Users typically find issues once they see the "real" data, sometimes leading to design changes.
Test database views comparing view contents to what is expected. It is important that users sign off and clearly understand how the views are created.
Plan for the system test team to support users during UAT. The users will likely have questions about how the data is populated and need to understand details of how the ETL works.
Consider how the users would require the data loaded during UAT and negotiate how often the data will be refreshed.
Regression Testing
Regression testing is revalidation of existing functionality with each new release of code. When building test cases, remember that they will likely be executed multiple times as new releases are created due to defect fixes, enhancements or upstream systems changes. Building automation during system testing will make the process of regression testing much smoother. Test cases should be prioritized by risk in order to help determine which need to be rerun for each new release. A simple but effective and efficient strategy to retest basic functionality is to store source data sets and results from successful runs of the code and compare new test results with previous runs. When doing a regression test, it is much quicker to compare results to a previous execution than to do an entire data validation again.
Taking these considerations into account during the design and testing portions of building a data warehouse will ensure that a quality product is produced and prevent costly mistakes from being discovered in production.
OLTP vs. OLAP
We can divide IT systems into transactional (OLTP) and analytical (OLAP). In general we can assume that OLTP systems provide source data to data warehouses, whereas OLAP systems help to analyze it.
- OLTP (On-line Transaction Processing) is characterized by a large number of short on-line transactions (INSERT, UPDATE, DELETE). The main emphasis for OLTP systems is put on very fast query processing, maintaining data integrity in multi-access environments and an effectiveness measured by number of transactions per second. In OLTP database there is detailed and current data, and schema used to store transactional databases is the entity model (usually 3NF).
- OLAP (On-line Analytical Processing) is characterized by relatively low volume of transactions. Queries are often very complex and involve aggregations. For OLAP systems a response time is an effectiveness measure. OLAP applications are widely used by Data Mining techniques. In OLAP database there is aggregated, historical data, stored in multi-dimensional schemas (usually star schema).
The following table summarizes the major differences between OLTP and OLAP system design.
| OLTP System
Online Transaction Processing
(Operational System) | OLAP System
Online Analytical Processing
(Data Warehouse) |
Source of data | Operational data; OLTPs are the original source of the data. | Consolidation data; OLAP data comes from the various OLTP Databases |
Purpose of data | To control and run fundamental business tasks | To help with planning, problem solving, and decision support |
What the data | Reveals a snapshot of ongoing business processes | Multi-dimensional views of various kinds of business activities |
Inserts and Updates | Short and fast inserts and updates initiated by end users | Periodic long-running batch jobs refresh the data |
Queries | Relatively standardized and simple queries Returning relatively few records | Often complex queries involving aggregations |
Processing Speed | Typically very fast | Depends on the amount of data involved; batch data refreshes and complex queries may take many hours; query speed can be improved by creating indexes |
Space Requirements | Can be relatively small if historical data is archived | Larger due to the existence of aggregation structures and history data; requires more indexes than OLTP |
Database Design | Highly normalized with many tables | Typically de-normalized with fewer tables; use of star and/or snowflake schemas |
Backup and Recovery | Backup religiously; operational data is critical to run the business, data loss is likely to entail significant monetary loss and legal liability | Instead of regular backups, some environments may consider simply reloading the OLTP data as a recovery method |
Data Mining:
Generally, data mining (sometimes called data or knowledge discovery) is the process of analyzing data from different perspectives and summarizing it into useful information - information that can be used to increase revenue, cuts costs, or both. Data mining software is one of a number of analytical tools for analyzing data. It allows users to analyze data from many different dimensions or angles, categorize it, and summarize the relationships identified. Technically, data mining is the process of finding correlations or patterns among dozens of fields in large relational databases.
http://www.anderson.ucla.edu/faculty/jason.frand/teacher/technologies/palace/datamining.htm
8 comments:
Very nice for beginners in ETL testing for Basics. Thank You Jai
valuable info ..thanx for providing
it's a Nice article, very helpful for us and thank's for sharing. we are providing ETL Testing online training
I actually enjoyed reading through this posting. Thanks for your posting , which helped me to join ETL Testing online training @ www.123Trainings.com
This is the knowledge that I had been craving for, I am already your rss reader currently and that I would frequently be careful for the new posts.. Thanks for providing the best information regarding ETL Testing online training
I got a good answer from the above description,but it still requires some more update to be made. Please share more content on MSBI Online Training Hyderabad
Nice and good article. It is very useful for me to learn and understand easily. Thanks for sharing your valuable information and time. Please keep updatingmicrostrategy online training
Thanks for sharing valuable information and very well explained. Keep posting.
etl testing online training
etl course
Post a Comment